The Future Of Pragmatic AI – A Conversation With Bob Rogers

Bob Rogers discusses the connection between artificial intelligence, logistics, and the significant impact it will have on our lives as we look at the future of Pragmatic AI.
In the dynamic dance of AI and logistics, we're not just predicting the future; we're orchestrating it. In this episode, Bob Rogers discusses the future of Pragmatic AI. He discusses the connection between artificial intelligence, logistics, and the significant impact it will have on our lives. Bob discusses the Nash equilibrium in AI, highlighting the inefficiencies that arise when we do not act together. He also touches on the difficulties of universal coordination, including trust issues and the practicality of companies accepting revolutionary ideas. But it’s not all that dark as Bob also shares the optimistic side of AI and the great potential it has for our future. Tune in now and learn what’s going on in our AI-driven world.
Links
We have Bob Rogers, who is an expert in artificial intelligence, on the show. As always, we are going to be talking about the present and the future. Bob has done a lot of amazing things in the artificial intelligence space. We’re very lucky to have him. I’m going to pick his brain about a lot of stuff that I see happening down the pipeline. Bob, welcome. Thanks for joining us. Tell us a little bit about yourself, how you got involved in AI and what you’re doing now.
Thank you. It’s wonderful to be here. I’m a huge fan of the vision for the show. I am very optimistic. It’s a great starting point. I’ll tell the story about myself going backward. I’m the CEO of a company called Orchestrated Intelligence that is bringing AI to supply chains in general because supply chains are struggling with lots of challenges around automation and efficiency. We certainly saw that in the pandemic when we couldn’t buy toilet paper, for example.
Prior to Oii, I was an expert in residence for artificial intelligence at the University of California San Francisco, where I led a team of data scientists who built the world’s first FDA-cleared AI on the X-ray device that was commercialized by General Electric as the GE Critical Care Suite. I’m extremely proud of it because it’s deployed all over the world and it’s saving patient’s lives in the ICU every day. I’m happy about that.
I’m also a member of the Harvard Institute of Applied Computational Science Board of Advisors, where they’re crafting a new generation of education around data science and AI for students out there. Prior to that, I was a Chief Data Scientist at Intel, where I lead Intel’s partner ecosystem for AI and analytics. Before that, I was a cofounder and chief scientist of a company called Apixio, which is a healthcare AI company that was acquired in 2020 by an insurance company.
Now, I’m going to answer your question, which is how I get started. I have a Ph.D. in Physics. I worked early on creating digital twins of supermassive black holes and other galaxies. My research was very computational in nature. I was working on Advanced Computer Modeling in the early ‘90s. One of the things that came across my desk was this idea of an artificial neural network, computing the way the brain computes as opposed to more traditional, very rigid rules-based computing.
That fascinated me. I added artificial neural networks to my research program and collaborated with some great folks. Part of that, in ‘93 I wrote my first book on artificial intelligence called Artificial Neural Networks: Forecasting Time Series. In the early ‘90s, neural networks were very limited by computing power and access to data. We solved some interesting problems, but it wasn’t quite enough to catch fire.
Fast forward 20 to 30 years, all of a sudden, we have Modern AI convolutional neural networks, computer vision, and these days, ChatGPT and all the other deep learning models. The world has exploded in terms of our ability to use AI, but the underlying tooling has dramatically changed as well. What started as an intellectual curiosity has become a guiding light for my career.
It is certainly a question and very significant to be in this field. With the advent of ChatGPT, everybody knows and everybody’s excited about AI. The first question that I want to ask you is, is it an existential threat because that’s what everybody thinks about? Looking at this from layman’s Terms, personally, I don’t think it is. I think that it’s going to be a great future when we all have our own personal assistant who’s able to do a lot of things. I always talk about the admin work of being a human in 2023. It’s too much. I’m overwhelmed by all of the stuff and to have somebody that can do with the monotonous stuff, for me, would be a godsend. How do you feel about it?
I agree with you. I always like to talk about AI as Augmented Intelligence rather than Artificial Intelligence. Most of the tooling that I built in my career is helping people do the more interesting work that humans are good at and automating away the horrible, tedious stuff that humans aren’t good at. There are all these types of roles I’ve seen in academic medical centers, for example. I’ll give you a very specific example. At UCSF, we receive 1.4 million faxes per year. Did anybody even know the fax still exists?
They definitely exist in the medical community. I’m a first-hand contributor to that problem.
A contributor and probably a victim. Those 1.4 million faxes are a whole variety of different types of documents. They would be reviewed by a human. They would be put in a queue to be reviewed by another human. Quite often, five different humans would touch those faxes before an electronic workflow was created.
That is a huge cost in terms of human effort. It’s not good work to look at a fact to type in somebody’s name, and where the facts came from is a horrible word. Nobody likes that word. Furthermore, it adds noise and errors to the information stream. Here, you have this nice, crisp piece of information. It’s not any great format because faxes don’t tend to be a great format for electronic applications, but then you start having humans transcribing and, unfortunately, making mistakes.
There are many reasons not to have humans need to do that. At UCSF, we built an AI pipeline using a combination of the latest large language models and transformers along with computer vision to allow us to understand not only what was written on these documents but also how to contextualize it based on little boxes, the boxes demographics so now I know that this name in this box is a patient’s name.
It was this nice marriage of computer vision in large language models. It didn’t allow us to automate, identifying what this document is about, who it is about, where it should go and then going straight into a pipeline. The impact of that for the patient is that it allowed us, in many cases, the dramatically short time it took to schedule a patient with an urgent condition because you can imagine all those manual steps delay the process. A beautiful example of augmented intelligence. Nobody is being replaced, but the primary part of their job is being automated so that they can do the part that’s more interesting for humans.
Let’s double-click on that for a second because I feel like that is something that a lot of people and different industries would benefit from. UCSF is a large organization that probably has the resources to bring somebody in like you to develop this for them. I worry that artificial intelligence is going to be another competitive advantage for the people at the top. There’s not going to be as much democratization of this powerful tool for your small doctor’s office. Is that fair or is that unlikely as the scenario?
A few years ago, looking forward, one would definitely have that concern, think about how much even basically developing the foundational capabilities, ChatGPT and some of the other larger AI models that started the whole revolution of large language models. Those were pretty strictly the purview of large Cloud companies that could access billions of records of data and have the computing and financial wherewithal to turn them into AI models. What I think has revolutionized the language model is something we call transfer learning, which is the idea that model trains to do one task. When I say training, I mean showing it data and examples of what you want it to do. It teaches you how to do it.
What may have really revolutionized language models is something we call transfer learning.
In transfer learning, you take a model trained on one task and apply it to another task. You give it a smaller number of examples, in other words, less cost less, computing and it can do that test very well as well. The first exciting example of transformation happened with computer vision when computer vision algorithms between 2009 to 2012 were developed to recognize cats, dogs and motorcycles in photographs. Those same models were usable as starting points for doing even medical image analysis. UCSF used some of these early very basic classifiers to build technology that went into the critical care suite.
Transfer learning allows you to take something that costs a lot of money to build, a lot of computing and a lot of data and then use it on another problem with a minimum of effort. Large language models ChatGPT has taken that to another level where you can type in a prompt and then get a very good answer to a problem that ChatGPT wasn’t necessarily explicitly trained to do.
The point of this whole Exposition on transfer learning is the ability to create chunks of value that organizations, individual practices, etc., can use. You’re not going to develop yourself, but the financial barriers are not so high that there will be vendors who create these technologies that make it easy for us to use in our smaller real-life applications. In other words, I think that transfer learning allows us to have access to many powerful applications that vendors can provide to us at a low cost and that’s going to democratize a lot of the capability. We won’t see that huge stretch between the halves and have lots.
I hope that’s true. One of the things that excited me about artificial intelligence, but the reality of what I’m reading in the news is becoming less and less what I see is the idea that you can use existing models to build your own software. Whereas a few years ago, a team of 6 would take 3 months to make something. Now, they do the same thing with a team of 2 and it might take you 2 weeks. Is that something that is accurate? It’s something that I’ve heard from other artificial intelligence experts on this and they’ve said this thing to me. I haven’t seen a trickle-down into reality.
I think it is true. I can give one example. This is something that we’re doing at Orchestrated Intelligence. One of the ways to visualize supply chain information is on a map. You can see, “Where’s my inventory risk or my service risk? Were my costs going to be a problem on the map?” What we’ve been able to do is connect a ChatGPT-like interface to that to allow people to ask questions about the data because underneath the hood is this giant wall of data about the supply chain that’s been computed using our our technology.
It’s very hard for people to get information out of the giant brick of numbers, but when they can ask a natural language question and then it surfaces the answer to that question in graphics, that’s very powerful. If we had tried to do that a few years ago, it would have been three PhD students for a year and a huge amount of funding to have that capability to say, “You’re going to type something and then we’re going to be able to show you the graphic display that goes with that.”
It’s very hard for people to get information out of the giant brick of numbers, but when they can ask a natural language question, and then it surfaces the answer to that question in graphics. That’s very powerful.
Now, it’s literally a couple of weeks of work for a couple of engineers. I do see in a lot of cases that we’re able to quickly create at least basic applications with these tools very quickly. I have a friend who works in child safety. He’s developed a number of amazing tools for law enforcement agencies to use that have taken years to build and now he’s been able to build on over the course of six months that are pushing the envelope of what you can do to prevent child safety challenges on them. I think it is real.
It’s something that people keep talking about. I haven’t seen a trickle-down yet because I do think that to truly feel like the future, we have to have software penetration into everything, an excellent, thoughtful user interface for everything, whether it’s my smartphone, a pump at the gas station or whatever it is. The public gas station has been tried and true for decades, but that’s my introduction to people about how software affects their daily lives. I feel like a creation of that software. For a long time, it’s been slated towards people who have the resources to build that. I wonder when that’s going to be something that’s available to all of us.
I’ve seen videos of people who are using it to code and everything like that, using language models to help them with coding. I’ll make it more efficient. I feel like I don’t know enough about AI other than large language models and then the idea of the visual representations being correctly sorted, like what you had talked a little bit about about the imaging for radiology and stuff like that. I could understand artificial intelligence to be a huge help, but there are many different interesting edge cases that I feel like all I’ve seen is this one main thing.
I will say that in my discussions with executives in large companies, one of the biggest challenges that they’re facing is that to build and adapt AI tooling, you need data and data from a number of different points of view. First of all, you need access to data across an organization or at least across a use case. Quite often, organizations have weird siled controls over data, which make it challenging, and then there are concerns about, “What is the quality of our data?”
One of the biggest challenges that businesses are facing is to build and adapt AI tooling.
Honestly, I will say that most organizations are worried about the quality of their data. Those fears are overblown. Data is decent enough using AI to patch over the parts that aren’t perfect. The other piece that’s challenging is quite often because data has not been a first-class citizen in most organizations. Sometimes, you’re not collecting the data that you need to collect to be able to do the next thing. It’s not so much that the data isn’t in the right warehouse, accessible or clean enough. It’s that you haven’t collected it.
One of the things I knew about Amazon early on was that the absolute principle for forming and building infrastructure at Amazon was that everything had to be measured. If it’s not measurable, then we’re not going to pay attention to it. If you want something to be important, you’ve got to be measuring it. That’s given them a huge advantage in terms of instrumenting their entire end-to-end ecosystem in the whole buying experience. Many organizations are not measuring all the little bits and pieces that they would like to have to instrument. Access to data has been a challenge, but I think it’s getting better because everyone’s thinking about that. I’m trying to figure out how to get better at capturing what is going to be relevant for specific problems.
Especially with your business being in logistics, I feel like there are many intricate pieces to logistics. There are any data points that are going to be interesting to see once it’s all a symphony as opposed to a bunch of different bands playing together. That’s going to be something interesting to see. Science fiction is against my inspiration. One of the action movies, I, Robot with Will Smith, came out and they have this highway.
There was one lane that was only semis. I can imagine that being the logistics or super highway that eventually, when we can eventually make all of these different intersecting companies work together, then it’s going to be like a main artery versus all these little capillaries in my field. That’s something that excites me. I look forward to the convenience of that. What excites you about it?
I love the idea of making things work better the way we expect them to. The trick on the logistics and supply chain side of things is that the supply chain lacks automation. It’s very reactive. What happens is there are people who are getting on phones and calling the factory or calling UPS and putting a palette on a plane to make up for the fact that the supply chain has strict somewhere and the product isn’t going to get there in time. You touched on why we call it Orchestrated Intelligence because we think that there should all be a coordinating symphony and not a cacophony of reactions to events.
The way supply chains work nowadays is you have some rigid handwritten rules that determine when you’re going to order more of whatever it is you need from your supplier. Those rules sit there and hopefully get triggered in time, but they’re not reactive at all. They’re not proactive. They’re not predictive. They’re just waiting for the world to happen to them. I love the idea of of taking AI tooling and automating the steps in front of that to make that all work well, giving organizations control over their costs, services and inventories.
More importantly, taking out that not-productive piece. Calling up the factory and saying, “You’ve got to stop production and make this other thing because we’ve got a problem,” is not helping any of this be more effective or more efficient. It goes back to our thing of that’s not the interesting human thinking that you want to be doing. It’s this task you have to do, whereas figuring out where you think the next changes in your demand and customer experience are is what’s more interesting. I do like that Automation and making things work the way you think they should work as opposed to being very reactive.
I think that’s going to be an interesting thing to see, especially with all these big giants like Amazon. They’re geared for this technology. I can’t even imagine stuff getting to me any sooner, but I want it to be coming sooner. If I had the option of 1 hour versus 1 day, I would use 1 hour every time, which is artificial intelligence. There are too many variables for human beings to be able to coordinate.
One of the things that you talked about, which I thought was interesting before we started talking on the show, is the idea of AI protecting your privacy, which is another huge benefit of AI. I feel like part of the admin work that’s required with being human in 2023 is not only remembering passwords and everything like that but accessing those passwords and being diligent about making sure that they’re not put into the right places.
I feel like that is something that I look forward to as some sort of artificial intelligence. It’s able to not only just be an encryption source but also advising, “This is out there. This password is out there in the dark web. You should not use this password. Let’s switch to another password,” and then we’re going to start using it.
Going back to my colleague’s tooling for child safety. They’re starting to get good at building AI that can go out and crawl and look for, “This is a risk. This is a pattern that’s not good for you,” or indicates something. It’s not trying to predict that someone’s about to do something bad. It’s about the real patterns out there of activities that we don’t want to see.
It’s interesting you mentioned security because one of the things in my current life that I didn’t mention is that when I was at UCSF, one of the other things I did, along with a couple of other cofounders, is I wrote some patents around privacy and security for building AI on private data. That has been spun out by UCSF into a company called BeeKeeperAI.
The idea of BeeKeeper is that you can create an algorithm that needs to be trained or operate on private data like patient data, sensitive government information, or banking information for anti-money laundering. It can build an algorithm, starting with a basic framework, and then the algorithm goes to the data, so this private data never leaves its origin. It gets computed, or the training happens. You don’t see the data. You just know that your algorithm is doing what it needs to do.
The idea of BeeKeeper is that you can create an algorithm that needs to be trained or operate on private data.
You can do that across multiple data sites, which would be Federated training or deployment. The data stewards, the ones with the data, have this safe infrastructure for your code to run without any risk that the data escapes, but at the same time, they can’t see your algorithms. You maintain the privacy of your algorithm, whether it’s for commercial reasons or maybe it’s national security. You don’t want the bad guys to figure out how to avoid money laundering algorithms.
All of that ability to enclose computations within a secure environment is very new. I think it’s exciting. It solves the problem that you alluded to earlier. Think about that super highway for trucks where people are coordinating their activities so that we’re much more efficient. The average truck in the US is 40% full. From an ecological point of view, that’s a disaster. From a financial point of view, that’s a disaster. How do you fix that?
One of the ways to fix that is to have, “My company needs to send this 40% of its truckload now. It’s got to go from point A to point B.” Somebody else, I guarantee you, has another 40% that needs to go to the same place at the same time, but I don’t know who it is because we’re not coordinated. The biggest challenge to coordinating that information is privacy. I don’t want anyone to know what I’m telling to who and what shipments are.
I have a weakness. Maybe it’s just information about my customers. I’m not saying this is something BeeKeeper will do, but in principle, this security around AI and data where all the computing happens in secure environments is going to absolutely change our ability to bring things together and coordinate. I’m excited about that because that’s a perfect marriage of technology with psychology. Whether or not our security fears are real, we are very nervous about our information being shared. Technology that makes it possible to coordinate without sharing is very exciting.
Are you familiar with the Nash equilibrium?
Yes.
The idea, for readers, is that when not acting in concert with each other. There are a lot of inefficiencies in the system, but if we act in concert with each other, then everybody can play to the highest level. I tend to agree with that. I feel like what I see is a detractor from this adoption of some of these ideas of universal coordination, which I want to happen, is the idea of all of the companies buying in.
For example, in BeeKeeper. It’s a great tool, but they need all those other companies to give legitimacy to BeeKeeper and say, “You’re going to be the steward of our data.” I want that. I want the concierge and AI to protect me so that my data is not lost. Do you think that it’s realistic that people are going to buy into this?
I do and I’ll tell you, there are two things. The first one is, in the case of BeeKeeper, we isolate BeekKeper from the data. You don’t have to trust anyone except for the very testable encryption and security protocols that wrap around it. BeeKeeper doesn’t see anything. Algorithm developers don’t say anything. Data storage doesn’t say anything.
That does take away one of those barriers, which is, “That’s all well and good, but do I trust BeekKeper? Is everyone at BeeKeeper who has access to the infrastructure trustworthy?” That is a big leap. We agree with that. BeeKeeper has been very carefully designed to be truly zero trust from all three sides. I think that helps. The other thing is you remember this from your own life in healthcare. There used to be a lot of work and money around healthcare information exchange. The government set up some big projects to exchange data in these hubs for the benefit of having more access to data to care for patients.
You knew that a patient had, in some other healthcare organization, an allergy to penicillin. You wouldn’t give them penicillin, but in the absence of that information, it’s harder. The idea was very good. That was a case where you definitely couldn’t get everyone to buy it. One of the reasons was how much money you have to spend to get your data into a format that everyone else could do. It was enormous. It was impossible.
If you transfer from one hospital to the next within a 30-mile radius, all of them are different data servers, and all of them have different sets of data. I see that as such a huge roadblock to all this coordination. If you like, healthcare had this interesting case example for all these other industries because we became digital a couple of decades ago and the day that we have now, a lot of it is unstructured. It’s good for radiology and these specific cases, but privacy is such a big task.
It’s an obstacle. I 100% agree with you and in fact, you know expanding that from healthcare to enterprises is large. When I was at Intel, I can’t tell you, this number is in the hundreds of companies. I saw doing huge multi tens of millions of dollars data lake projects, “We’re going to put all our data into a data lake. We’re going to transform it and harmonize it.” They spent truly tens of millions of dollars at least. At the end of the day, The CEO would ask, “What’s the ROI on my data lake?”
If we spent $20 million, then your Roi is negative $20 million. The reason is you’re doing all this transformation, infrastructure and technology that you don’t have an end objective in mind. They had a fantasy that the insights would bubble to the surface of the data lake. Here’s what I’ve seen that does work and where I hope that industry, healthcare and supply chain are evolving is if you start with a single problem, here’s something I need to solve. It requires these pieces of data. They come from a few different sources, but when I saw this problem, it created this much value. I’m going to build a little bit of infrastructure. It takes connecting the dots there, and then I get ROI.
When I’ve done this project, the CEO asked me about the ROI. I say, “I saved $100 million because I reduced the inventory for my company across the board. This is a lightly veiled Orchestrated Intelligence use case, but it applies to everything. It applies to healthcare.” I always ask people this. Have you read Cat’s Cradle by Kurt Vonnegut Jr.?
I don’t.
It’s a great short read. There’s this concept called ice-nine, which is ice that freezes at a higher temperature than normal ice. If you drop a crystal of ice-nine into the water, it all freezes that little crystal. It nucleates a crystalization. The way I think about these small projects, bringing together the minimum information, is they create the ice-nine for the entire organization to start using data effectively. You start to see the patterns of what to connect and what matters.
As all my cards on the table, I’m the Founder of a surgical practice that employs 200 people and see all of these advancements happening and we’re still like very much like a mom-and-pop business and we do things the same way most people do things, but you see all of these advancements happening at artificial intelligence. You’re wondering where the direction is going and stuff like that. It’s nice to know the insight.
I’ve never been able to prove the ROI. It’s all my other cofounders. I’ve been saying for a long time, like, “We need to get a CTO. We need to bring somebody in who knows technology in such a way because I feel like if we can be at the forefront of this industry, it’s going to hold a lot of benefits.” I never thought that proving the return on investment from a small task would show them that would be something that we should start investing in. That’s an interesting topic. I like that. I’m going to take that.
Ice-nine is showing up everywhere.
I would be remiss if I didn’t talk to you. You’re in the logistics space. We’ve seen this trend of globalization making such an environment that China and other countries are where the manufacturing happens and the US is where other decisions are made that are not manufacturing. Artificial intelligence is going to certainly make everything more efficient. Is it going to affect the supply chain in such a way that manufacturing will be here again? Do you think that’s going to happen?
When they think supply chain, the majority of people think about all the issues that happen around COVID, about how we had this huge kink in the system because China was not available to us anymore. Now we’re having you know a lot of insight and a lot of people looking at this as a potential problem, but I feel like I don’t know if artificial intelligence is going to close the gap. I feel like humanoid robots are going to close the gap and that’s part of artificial intelligence, but you’re in this space. How do you feel about how the future is going to play out?
There are a couple of levels there technologically. Certainly, the pandemic and some of the challenges with the wars that have broken out. I can see enterprises rethinking their globalization strategies and pulling in, looking for smaller footprints for their supply chains to something that they can control more, supplies and companies manufactured in Asia coming from Asia become more of a concern. What we’re seeing at Orchestrated Intelligence is interesting. It’s the combination of the AI and better instrumentation.
What we’re seeing at orchestrated intelligence is the combination of AI and better instrumentation.
I don’t think people running supply chains have understood how costly long lead times are. It takes 30 to 60 days for something to cross the ocean on a ship. That has a profound impact on the overall performance of your supply chain and is even more hidden from people who are running supply chains. Something that we expose is the variability of that lead time. If you knew, like clockwork, that something was going to arrive in 40 days, then you could set your clock by it and your supply chain would run smoothly.
A lead time of 40 days, sometimes it arrives in 34 days. Sometimes, it arrives in 75 days. That uncertainty kills you. We’re working with one of the major shipping companies, incorporating their ability to shorten and reduce variability in shipping and showing customers how it impacts this by chain in positive ways when we reduce those variabilities. As that becomes more and more understood, it creates financial pressure to find solutions that have shorter shipping lead times and that becomes part of the equation. Quite often, supply chain is thought of as the cost of doing business.
It’s not seen as a knob that you can control and it’s all part of your overall story. That’s an area where AI and better analytics are helping. This is a general statement about AI. AI is very good at taking tribal knowledge or local know-how, mechanizing it and creating an ongoing evolving knowledge base. As we get good at tracking how different manufacturing, assembly and logistics processes happen, the AI will allow us to transfer that knowledge from one location to another. When the business case is, it might make sense and be easier to replicate an operation in closer to home than elsewhere. Knowledge transfer is aided by AI, probably the way to say it. That’s an important point.
The universal translation alone. My daughter, at two years old, watched these cartoons that are obviously made in some other countries. The culture is totally different in these cartoons, but they’re speaking in English and it goes both ways. The best ideas that we have can easily be transmitted to other cultures as well. I feel like that is interesting. The internet was the first step in this. We’re still based on our country or language of origin. That’s something that artificial intelligence is going to help with. I’m excited about that.
It’s way more ability to connect and translate. That knowledge transfer piece is huge. That’s a big one. You mentioned robots, which I’ve observed is fascinating in the logistics world. I’ve been going to logistics, warehousing and supply chain trade shows for a number of years now. Early on, there were good robotic systems. Think of both picker arms, like an arm that can pull apart and put them into a box like, “I need a B and D for this one. B, C, E and F for this one.”
Humanoid robots are moving around, taking stuff, picking and moving parcels around a warehouse. Those things existed a few years ago. They were starting to come down in price because the technology reinforcement learning and some of the other component parts have gotten cheaper. What I saw was executives thinking about, “I’ve got a choice between a capital expenditure to buy this very cool robot. It doesn’t require maintenance and energy, but it doesn’t require work breaks. There’s no shrinkage from theft in the warehouse or anything like that.”
On the other hand, it is a capital expenditure. I don’t totally know how this thing is going to last. Maybe it’s going to be a lemon. I have people who can do this job already. It was a toss-up. There was not a massive adoption of these things, but there was interest. In the last few years, the number of people who are willing to take warehouse picker jobs has fallen off the cliff. There’s been a huge lack of humans to do these jobs. As a result, people are buying these robots like they’re going out of style. It’s a massive flood of this AI-driven automation. Costs are coming down rapidly. Very few people’s jobs were being replaced. It was more like, “We can’t get this work done without this automation assistance.”
It does tie back to our original augmented intelligence in the sense that a robot’s job is probably not a super stimulating, healthy job for a human. Obviously, sometimes people still want their jobs, even if they’re not great jobs, but the flow of technology has been driven by the lack of people wanting to do these jobs rather than people being pushed out the door because the robots came in. I think that’s very interesting. That speaks to the transfer of knowledge because every time you replace a human, you have to retrain them. To retrain a robot, you literally copy and paste a piece of code into the system. Knowledge transfer is easier.
Who do you think’s doing it the best of all the companies? Who do you think is making the advancements and AI that are going to be most beneficial to society?
On the robotic side, companies like Boston Dynamics are the best at marketing. They create all these interesting. They’ve got a dog and a robot. They’re creating a lot of awareness. I would say that there are a lot of companies innovating and specific areas and they’re all bringing some value. On the big picture AI side, open AI certainly has led the way in terms of its mission, even though it may be struggling to carry it out effectively. Our mission is to create AI That’s good for you. I think that’s helpful because we do want that. People probably don’t know ChatGPT was originally based on GPT3, which is a large language model.
ChatGPT has a lair of control on top of it that was trained to make it be nice. It’s like if you ask it to tell you something bad. let’s take something simple like, “How do I build a bond?” ChatGPT will say, “I’m sorry. I’m not programmed to give you that information.” GPT itself has that information. They’ve created a layer on top that makes it human-friendly. That is an important principle for all these. They’ve done a good job.
I’m a huge fan of Satya Nadella, the CEO of Microsoft. He has both turned Microsoft around culturally and made great investments. I don’t know him personally, but with everything I know about him, I think he’s pushing things in a good direction. Microsoft is bringing a lot of nice consumable services to Microsoft Azure. It’s interesting. It this blows back in court, but in healthcare, it’s been a platform of choice for cloud computing. The other cloud providers also have a stake in that.
I’m a huge fanboy of Microsoft, specifically Satya Nadella, but in general, I bought my first Windows phone. I got the Tiles. We’re going to revolutionize the industry, and then like I’m talking to you on a Surface Pro. The entire Microsoft Teams and Office Suite are my exclusive. I don’t use anything else other than that, but regardless, Satya Nadella is the type of leader that I want to be. He’s intellectually curious. I hope that Microsoft molds these different disparate elements into something that’s providing a lot of value for humanity.
I think it will. That has been his goal since day one. We’re getting to the end of our time. I don’t want to miss these questions that I asked all of my guests. It was probably interesting speaking with you, but I do want to get your insight on more general stuff because, as a thought leader in AI. I do want to find out what your motivation was for doing the things that you do. For me, a lot of the stuff that I try to push across is because of the science fiction and Utopian fantasies that I was reading when I was a boy. I want to make sure that we live in a future that everybody can look forward to. The best for Humanity is yet to come, but that’s just me. What about Bob Rogers? Where do you get your inspiration from?
It’s interesting because I have a similar genesis. One of the aspects of my career that I didn’t talk about much was after I wrote my first book on neural networks, which is called Artificial Neural Networks: Forecasting Time Series. People called me up and asked me, “Can you forecast the stock market?” I researched it a little bit. I met a guy who had a trading firm. We founded a hedge fund that we ran for twelve years very successfully. It was something I fell into like, “I get to do modeling and data stuff. I can make money. This is cool.” Not to be too crass, but I wasn’t put on this Earth to make rich people richer.
I wanted to have my presence on Earth to have a positive impact for humanity. I left that field and switched to healthcare around 2006. The idea was that I was going to ply my craft around analytics, AI, and data science in a way that helps humans. When I mentioned the GE Critical Care Suite, I’m extremely proud of that because if you’re in an ICU somewhere in the world and they’ve got that system in place, if you start to develop a life-threatening collapsed lung situation, it will not only identify it in your chest X-ray, but it will alert the radiologists that they need to look at an image immediately.
It changes the game for people’s safety. I love the idea of building new things that cast a broader shadow of good on the world. That’s why I am super excited to come on this show because I think what you’re doing here is fundamentally saying, “Let’s not all be gloom and doom about existential threats and war and pestilence. There’s a lot of good. We have to all mold our part of it to move in the right direction.” This is the inspiration for that.
That’s why I got into healthcare. I’ve been enjoying the supply chain because there are fewer restrictions on applying the solutions and getting them out there. At the end of the day, when we improve a pharmaceutical supply chain, for example, people can be ensured of getting the drugs they need when they need them. When we’re doing food, it’s building resiliencies so that our food supplies don’t get disrupted if something crazy happens that we didn’t expect. I think that’s exciting.
I totally agree with the impact factor being a decision and why I do what I do, but it’s exciting to see that in real-time like you have the data in front of you. You can tell yourself, “I’ve improved based on this metric,” which is cool. The second question I ask is that other than what you’re doing, which is AI for logistics, what other technologies are coming down the pipeline that you’re excited about? IT could be an AI application like full self-driving, for instance. I always tell people I cannot wait until full self-driving comes. I’m going to be the first person online for full self-driving just so I don’t have to commute anymore. A 30-minute commute could be 30 minutes of Zen meditation.
I’m laughing because when I was at Intel, I used to give these keynotes to these huge audiences all the time. I would always talk about autonomous vehicles and ask the audience. There’s always a split in the audience. Some of them are like, “I will never take my hand off the wheel.” Sometimes, it’s a risk or a fair thing. Sometimes, it’s, “I want to be in control of my car. I like that feeling.”
There’s the other part of the audience that are more like, “I’m spending a lot of time in my car wasting valuable CPU cycles when I could be thinking about other things or meditating.” I’m definitely in the category that you’re in. I can’t wait to be able to sit there, think about other things, and not have to pay attention constantly. It’s a great example that you brought up.
I’m quite fascinated about quantum computing. I think it is becoming real. It’s interesting from a technological point of view because I talked to a technologist in deeply embedded in research labs and stuff. I’ve had some of them tell me quantum computing is 1 or 2 years away. Big changes are going to happen in our ability to compute certain kinds of things. Other people in labs have told me, “Error checking for quantum computers is primitive.” Maybe it’s going to be like fusion, where every 10 years, we’re 10 years away. That juxtaposition of outlooks among experts is fascinating to me.
The impacts of quantum computing could be very profound. There are some basic security things, such as it being true that quantum computers can break encryption. They are looking on the downside. There are roadstates that are collecting encrypted data, hoping that when they get a quantum computer, they’re going to be able to break it and find out secrets. That’s something to pay attention to, but it also speaks to the profound difference in the level of ability to compute. I think it will play a role in how AI evolves. There’s a whole other level of interesting ability for quantum systems once they come on board in a real way.
You’re the first person that said that. I honestly don’t know too much about quantum computing. I’m going to do a deep dive after this. I appreciate you enlightening me with that piece of technology. Last question, where do you see society in ten years with the adoption of AI? What is it going to look like for you? What are some things that we can get excited about?
You certainly hit on one, which is autonomous vehicles. A lot more automation in how we get around the logistics of our daily lives. I think that we’re certainly going to see jobs shift. As I said earlier, they’re shifting to we’re doing less drudge work. We’re doing less stuff that’s repetitive and monotonous. We’re being asked to do more mental things.
A colleague of mine, a4 my co-author for one of my books called Demystifying AI For The Enterprise, Prashant Natarajan, said something that I thought was very fascinating. As AI systems get better and better at rote tasks and things that involve memorization and repetition, what does a college education look like?
It starts to create a bigger emphasis on more like classical education. How do you think about things? How do you put ideas together? How do you synthesize, interpret and project? I think that’s a very appealing view of the AI future where we’re reading, thinking or putting things together more. The work is a little bit less ditch-digging and a little bit more architecture.
That’s something that I feel should be emphasized more now because we’re on the verge of this AI revolution. First principles thinking is what I tell all the people coming through my residency program or other students I teach. That is what you need to make sure that you understand because the information is out there.
Rote memorization is going to be a thing of the past. We need to be able to think correctly and find that information correctly. Especially since your background is in physics, I feel like that’s something that’s almost a dogma in the realm of physics. This was interesting. I feel like we learned a lot from you. Thank you so much for sharing your wisdom with us. For those of you guys who are reading, please like and subscribe. For those of you who are reading on a regular basis, we will see you in the future. Have a great day, everybody.
Thank you.
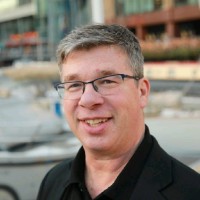
Bob Rogers
Product Leader | Co-founder
Bob Rogers, PhD, is CEO and Co-Founder of Oii.ai. Previously, he has been Expert in Residence for AI at the University of California, San Francisco, and a member of the Board of Advisors to the Harvard Institute for Applied Computational Science. Bob was also Chief Data Scientist for Analytics and AI at Intel, and was Co-Founder and Chief Scientist at Apixio, a healthcare AI company that was acquired by Centene in 2020.
Bob began his career with a PhD in Physics, developing digital twins of supermassive black holes in other galaxies. In 1993, seeing the future potential impact of AI, he expanded his research to include artificial neural networks, the progenitor of modern AI technology. He co-authored the book, “Artificial Neural Networks: Forecasting Time Series,” which led to a 12-year career as Co-Founder of a quantitative futures trading fund. He received his BA in physics at University of California, Berkeley, and his PhD in physics at Harvard. He has been featured in numerous publications including Forbes, Inc., and InformationWeek.