The Future Of Clinical Research - A Conversation With Brigham Hyde

Brigham Hyde, CEO of Atropos Health, talks about the future of clinical research and how they are bridging the evidence gap in medicine.
Many people don't realize that a lot of medicine is detective work. Every decision is based on the evidence available to you. However, there is an evidence gap in medicine today, making it difficult for those in this space to provide the appropriate care patients need. Brigham Hyde is bridging that evidence gap through his company, Atropos Health. Using millions of anonymized patient records, they help providers answer clinical questions that have fallen through the cracks of the evidence-based literature. In this episode, he shows us the bright future of clinical research as they provide a way to relay data to physicians by harnessing the power of user experience and automation. He dives deep into democratizing information and affecting change to create better patient outcomes. Learn more about the technology behind Atropos Health and how they are changing the world of medicine through clinical research. Let Brigham show you the bright future ahead!
Links
-
Aubrey de Gray – Previous Episode
We are talking about the future in the present with Brigham Hyde who is the Cofounder of Atropos Health and also a thought leader in the intersection between data and healthcare. Thanks for coming, Brigham. Tell us a little bit about what you’re doing at Atropos Health.
Thanks for having me, Dr. Awesome. Atropos Health is a company I cofounded with Nigam Shah who’s a Chief Data Scientist at Stanford and Saurabh Gombar who’s our Chief Medical Officer. It was a spin-off of technology that was developed there. Originally, the technology was called the Green Button, a simple idea, “Could we give physicians at the point of care a second opinion from all this great healthcare data that we have collected?”
The key to doing that was making it a very simple user experience. Send us a couple of sentences like you’re emailing a colleague, “A patient looks like this. Drug A or drug B, what’s better?” We would respond within about a day with a full boat observational research study as they would read in a publication based on millions of patients in the EMR that look like that patient.
It’s truly personalized medicine. We deliver back is an eConsult. A physician on our side reads the results, writes a summary, and talks them through the findings, and then the physician can choose to factor that into care decisions. It’s funny to say this, but if you ask most people, “Does my doctor look at millions of patients like me when they make a treatment decision?” the answer is, sadly until us, they don’t. There are a bunch of reasons for that, but this enablement of that type of insight back to clinicians is leading to an amazing impact in patient care, outcomes, and even how health systems can run and operate.
It’s also driving forward research. Our fundamental belief is that medicine phases what we call the evidence gap. It’s a simple problem that we all understand, which is that there are not enough clinical trials to show evidence for every decision that needs to be made, and the clinical trials that do exist tend to exclude most patients, whether we’re talking about those with chronic comorbidities who are systematically excluded from trials yet make up in the US a big part of our patient population or other demographic groups that are leaped out of that process.
We think there’s a big issue there because physicians like yourself have to extrapolate every day from imperfect evidence, “This trial looks like my patient.” We’re saying, “You don’t have to do that. Let’s give you a way to get evidence directly on patients like that.” The key to that is great user experience, automation, and a lot of rapid turnaround. That’s what we do.
Many people don’t realize that a lot of medicine is detective work similar to Sherlock Holmes. You make your best guess based on the evidence that’s available to you. For example, I’m on call. I had a patient with necrotizing fasciitis, which is this flesh-eating bacteria. Requirements are surgical drainage. You put them on antibiotics, and the antibiotic regimen is like, “You could use this or this.” I’m like, “Which one do I do here?” There’s no randomized clinical trial for flesh-eating bacteria because it’s so rare.
There’s a randomized clinical trial on each one of those antibiotics, their safety profiles, and everything like that, but the actual application of that medicine is not as studied other than not necessarily anecdotal evidence but evidence-based, “I know that this bacteria causes this, and this antibiotic affects this, but how can we understand how one antibiotics is more effective than another?” When I was reading about you, I was like, “This is the gap that you’re talking about.”
Let’s take your example. It’s something we think a lot about or are obsessed about, thinking about our users and physicians and how they go about things. In your case, you’re looking at that list. You’re like, “There are five to choose from. Is there a guideline? Is there a trial that has been published on one of these?” If you dig in deep and do your homework there, you might find there is a trial, but it was on 150 people in the UK, and it wasn’t right. When you dig in behind the evidence, it starts to get pretty thin.
What do you do next? Maybe you would go to something like UpToDate. A lot of physicians do that. My wife is a physician. She is a heavy UpToDate user trying to stay on top of the latest. That will summarize what’s out there, but what if there’s no good conclusion? Usually, what folks like you would do, and tell me if you agree, is you might contact a peer. You might say, “Dr. So-and-so, you’ve seen a lot of these patients. What would you do?” What’s happening there is we’re trying to rely instead of on millions of experiences. Maybe this person has seen twenty of these patients.
Dr. John Halamka who’s one of our partners at Mayo Clinic likes to say, “I’ve never met a physician who can remember thousands of patients. It’s a fact.” You have people who are experts in certain fields, but they’re often hard to get to. You might not know the guy who knows that particular case better than anybody. We think of ourselves fitting in right between that UpToDate step where I went there, there wasn’t clear information, and I need to go talk to a peer.
We designed our experience to be like that, which is important because you don’t have time. You have to go to the next patient. You can’t sit there and type code or even have time to flip through a tool. You need a quick answer. You want to send off a note and get an answer back. That’s a key finding we have had. Think of how physicians seek information now and fit into that but add new robust information with a lot of transparency to how we’re doing it so that they can factor it in. That’s where we fit.
We think of how physicians seek information now, fit into that but add new robust information with a lot of transparency to how we’re doing it so that they can factor it in.
That’s interesting because the need is there. A lot of medicine is based on evidence, but the application of that evidence is trial and error. For example, if I put this guy on antibiotics and he gets better, then I made the right choice, but if he’s not getting better, then I have to look at it and be like, “Let’s reevaluate. What am I doing wrong?” That is not as precise as it should be in my opinion. It’s limited by time as well.
I have to wait for more information to come back. I have to wait for me to find out what bacteria is causing him to have this infection and how to specifically attack that bacteria. Let’s get into the nuts and bolts of it. How is this assessment happening? Is it through artificial intelligence? Are you looking at different research articles? How does the recommendation that comes from a platform like yours come about?
My cofounders, Nigam and Saurabh, began this at Stanford years ago. If you think about what I was saying about the user experience, we knew it had to be fast, clear, and transparent. It had to fit that mold of user experience. From a technology perspective, we didn’t build tech for tech’s sake. It wasn’t like, “There’s AI. Let’s do AI stuff.” It was all focused on meeting that need.
Everything that we built was focused on automating the process of doing what’s called observational research. Think of it as a registry. It’s even related to a clinical trial. It’s the same methods that are used in those trials, cohort studies, and other inferential statistical techniques, which clinicians are very used to. They’re trained to read those papers. They understand how to evaluate those statistical methods.
What we have done is that we have added significant automation from the data step to the answer step. Think of it like this. You have a health system that has been collecting electronic medical records for the better part of fifteen years now back to the HITECH Act and Meaningful Use. We have a lot of data, and it’s sitting largely in the cloud at these institutions behind their firewall. It contains useful things. It contains some more difficult things but mostly, we work on electronic medical records. This means we have the ability to analyze events that have happened to patients. It could be clinical events, labs, procedures, drugs, genetics, and sometimes other things like imaging.
We build a technology that is federated and cloud-based. Why is that important? It is installed within the health systems environment. People get concerned about data privacy and data possession, “What’s happening to my data?” We don’t take any data. We don’t buy data. We don’t sell data. This sits on top of that cloud deployment. We’re taking advantage of that evolution of the cloud infrastructure as well as the compute infrastructure you will hear about a lot these days.
Once we’re installed, we organize the data in such a way that makes it easy to analyze. If you’re doing observational research or statistical techniques like inferential statistics, everything is about the time between an event that happened to a patient, “How long before this happened? What sequence did this happen?” We organize data along a patient timeline.
We also do something called expressing knowledge graphs across that, “What does that mean?” That means I know what that thing that happened was. A diagnosis has an ICD code and terminology attached to it. A lab value has a LOINC code, and we have a value attached to that. We can read the results that come in through that. Everything is organized and standardized. That’s step one. Step two is that most of the time when people try and do this work, they have to hire a programmer, somebody who knows how to write SQL or Python. They’re writing lines and lines of code, usually several hundred per query, to pull out the patient’s set that most appropriately matches the question.
Let’s say we were looking at heart failure patients with a history of diabetes of a certain age range who had been on a certain medication. You have to write all that code. That’s normally very lengthy work, and it takes a while to do it. A lot of times, the person who is writing the code is not a medical expert. How are they going to define something like the history of diabetes? Is it that they had one diagnosis of it? Is it that they’re on insulin? How do you define these things?
We have a way of standardizing all of that. A non-technical person, usually a clinician on our team, uses our tools. Our platform is called Geneva OS, which stands for Generative Evidence Acceleration Operating System. Think of it as low-code Mad Libs for these queries. A physician can write a couple of sentences with very understandable and standardized definitions and then hit run. It pulls all those patients together and then automates the statistical pipeline.
Usually, after step 1 and step 2, somebody has to go in and do a statistical analysis, and you’re hoping they do it the best way they can. You’re hoping they correct confounders in the data. We don’t want to compare. One arm of patients is all 65-year-old smokers, and the other arm is all 35-year-old triathletes. You don’t want to do that comparison.
We do techniques like high-dimensional propensity score matching, IPTW, and others, which are the best-of-breed approaches to remove those confounders but all that is automated. Some positions sat down on our side, wrote a couple of senses of code, and hit run. That will produce a full study. They will read that and say, “What was the P value? What were the confidence intervals?” They will write a summary that says, “This is the conclusion of this study.”
That gets sent back to the clinician. It’s a report that can be appended to the EMR and shared with a patient if they want to explain to them why they’re doing something. Many of them go on to be published. We have produced over 50 publications this way, and many more coming. We have a 100% peer review success rate. All of our methods are published. You can cite them and see how we do everything.
Doing all that automation allows us to do a study. I’ve been doing this type of work most of my career. Normally, it would take months to do, and that’s the standard. We’re doing it in less than a day. Being able to do that makes it possible that a position like yours could leverage this if you had a question because you don’t have to wait weeks and months to find the answer. You can find it out now.
It’s also personalized. You can take dimensions about that specific patient. Maybe it’s their age, their history, or the treatments they’re on. Factor the analysis so that it’s just on those patients. Oftentimes, we end up producing the largest study ever run on that patient population every time we do this. You think about a topic like diversity, equity, and inclusion in clinical trials. You think about patient populations. You see they’re not well-represented. This is the answer for that because you’re going to run it on patients like them.
The application in my mind is very specific but some of what you said could be applied to much larger questions. Let’s say someone loses their arm. What is the likelihood that it is something that’s going to lead to premature death? It’s a longitudinal study like that. A lot of what you’re talking about is helping clinicians make better decisions but my question to you is this. Can this also be used for epidemiological studies to help prevent some of these downstream effects?
You should send in the question you described. We’re happy to provide an answer for you. Being on the show, you get one if you would like. We do that type of stuff all the time. That’s a very typical use. Sometimes a clinician might have a question like that. They’re trying to understand something. You can imagine that an answer to that might influence a policy on how to remove the whole arm, “What surgical approach do we take?” It gets factored into policy decisions a lot now, “Why do we always do it this way?” It could be a drug choice, a surgical choice, or a policy-of-care choice, “Maybe we should do this test.” We get questions like that all the time.
The other big area where you see questions like that is coming from the research side. This is a supercharger to research, whether I’m an academic physician with a field of research writing grants. We work now with life sciences companies and med tech companies to help them do their early stage research. A lot of times, that starts with epidemiology, “How big is the problem? Is there a problem? If there is a problem, is there an opportunity to treat it?”
I’ll tell you one example that came up. It got published. A professor at Stanford, Dylan Dodd, is an academic researcher. He studies the gut bacteria. He developed a mouse model and was NH-funded where he would test how changes in the gut flora or the different mix of bacteria end up influencing other inflammatory diseases. One of the ones he worked on was gout. What he found was depending on the mix of the gut bacteria, there are different levels of uric acid that get produced. The bacteria themselves are involved in that production. If you have high uric acid, you develop gout.
He proved this in his mouse model and started experimenting with different therapeutics he thought might shift the gut flora or antibacterials. He gave some of the mice clindamycin. He gave some of them Bactrim. These are well-established drugs that have been approved for years. He found that when he gave them Bactrim, it shifted the gut bacteria in such a way that it stopped the gout. When he gave them clindamycin, it made it much worse, which is very typical of what you see in gut bacteria shifts.
He’s ready to publish but he was aware of Atropos’ service at Stanford. He called us up and said, “Can you run a question for me? Can you tell me if anybody with gout in the data happened to be taking Bactrim or Clindamycin? Did they get better or worse? In addition to that, can you tell me if the rate of people getting gout is lower in those who happen to be taking Bactrim?” Nobody is prescribing Bactrim for gout at this point but some people with gout are probably taking Bactrim, and some people taking Bactrim get less gout.
He asked us those two questions. There was a blaring clear signal that he was right. I’m talking about tens of thousands of patients. Those who had gout and took Bactrim tended to get better. Those on Bactrim tended not to get gout. With Clindamycin, it was the reverse. We produced that analysis about a day after he spent years in the lab developing this model, and it confirmed what he was finding on the bench in real patients. He got into his publication. We became figures in the publication, and that has now been published in Cell. It elevated his research to an even higher level.
Let’s take a step back for a second. What that is saying glaringly clearly is that we should be giving back to the gout patients, and nobody does that. I still think clinical trials are critical. Maybe in this case where we have a drug that’s relatively safe, and it has been shown before to be, there should be an accelerated pathway to that but why are we not seeking a label for Bactrim to treat gout? That’s what that research says. That type of acceleration and using data to confirm what’s there is super exciting. I have more stories like that but I would love to hear your response on that one.
What made my brain explode was the expediency of getting that answer. I tell people this all the time. It’s my personal opinion, and you’ve been on both sides of this. You’ve been on the academic side and the private industry side. True change is not coming from academics. If you want to change the world in academics, it takes you decades. It takes you a long time to sit in the lab and pipet.
I pipetted. That’s how I started.
One of the things I know about you is that you’ve experienced translational research before you made the switch to the industry. Everybody that I talk to both in academics and the industry feels the same way. They’re doing this thing but if you want to change something, it’s through the industry. I always felt like there was a big disconnect because there’s this idea that when you transition to the industry, you have to make something profitable and effective but I never thought that there was a space for intellectual queries. That’s something interesting because there’s so much data. Something you highlighted is there’s so much data out there.
Let me tell you a personal story about this and a little bit about why I’m doing this. In undergrad, I was a chemist. I was making molecules. I got a chance to work a little bit in the pharmaceutical industry as an intern. I loved the science. I wanted to go deeper. I went straight into a PhD at Tufts where you’re a professor. My PhD is in clinical pharmacology. Most people with that degree go on to work for the FDA or design clinical trials. I was into the basic science. I worked on mitochondrial biology, stem cell development, and interesting work around hematology. I loved it but I felt that it was slow.
I could work on this one receptor for a decade. Maybe we will find something out. Maybe there’s a drug developed that helps people in the future. At that time, this is in the 2000s in Boston. It was the beating heart of the genomics revolution. Apologies to Stanford and other places. You did great work too but in Boston, it was so vibrant there with people like George Church and heroes of mine in that area. Seeing that, what I felt was there was going to be this wave of data that’s going to show up, and that data may contain more answers than I can produce pipetting one tube at a time.
I focused a lot of my efforts on that and began starting companies at the time. I have been chasing that the whole time. In between then and now, I’ve built a number of data companies like ConcertAI, which works in the oncology world data space, and Decision Resources Group, where we amassed large sums of data, claim side and also clinical. EVERSANA is another one. A lot of the time, in those businesses, our goal was to aggregate clean up and make this data useful because at the time, it was hard to find, and when you found it, it was a mess. Take that to pharma companies to help drive R&D.
That was great. A lot of great work was done through those companies and the value we created for those customers. I truly believe it helps advance R&D if you’re able to bring rapid data insights and not have to wait every time for a clinical trial to find something out. I used to have this recurring nightmare where I had access to a giant data set, this abyss of data. The answer cure for cancer was in there, and I wasn’t asking the question. I had that feeling for a long time.
When I got to know Nigam and Saurabh and what they were doing, I’ve been obsessed with it since I first met them because what if we could get to the questions? Doctors like you and other physicians observe things, have questions, and have no way to answer those questions without a lengthy research process. What if we democratize the question-asking? What could that do? The best part about working in this company is every single day, there’s a new question that comes in, which is going to have a massive impact on a patient. It’s so exciting.
Our job as businesspeople is to create a business that can allow that. I could talk more about my perspective on how you build these businesses. We have great investors with a long-term view. This can have a big global impact. If you think about my path, my curiosity has driven me to this point. It has been about trying to get to this because I’ve been trying to get the data organized and wait for the data to show up. It’s there. What can we do with it? Our timing is good for this given what’s going on in the broader AI space and all these things. We’re now able to think about getting to the questions. It’s not just getting the data.
It’s in the public consciousness now. The idea of data being this new gold rush has been out there for at least since Cambridge Analytica had all of their stuff that was publicized very clearly but I look at it as, “If this is a gold rush, there’s a lot of coal, dirt, and sand that you have to get through to get that vein of gold.” The hard part for me is that if I have a clinical question, I have to set up years of my life to answer this. It’s not something that is a very easy task, and it’s so easy to become complacent and not pursue that. It’s so easy for me to practice, do my thing, and not have to worry about these clinical questions.
There are great researchers here in Boston who are doing amazing things but the real excitement is coming from the industry. For example, you mentioned genomics. Longevity and life extension were a natural byproduct of that. The idea that we can extend life is so popular. I live a few blocks from this Marriott Hotel where they had a conference on longevity, and it was so popular that one of the guys was stating that Yamanaka factors are the answer to reverse aging.
This place was so popular that the conference that he was speaking at was standing room only, and then they had to call the fire marshal in to clear out all these people who were trying to cram in a Japanese subway station, trying to hear what this guy had to say. There is a lot of interest in translational research, molecular biology, or whatever it is. The tabletop research is interesting but it’s only coming from the industry side, which is interesting to me because growing up or going through my career, I always thought academics was where it was at, “This is the way to answer these big hanging questions that we have,” but now, I see it’s the opposite. Do you feel that way?
Longevity is a whole topic. My PhD work was in mitochondrial biogenesis, which is a lot of NMN and mitochondria. I’m like, “Mitochondria is back. I love it.”
I had Aubrey de Gray on. He thinks mitochondria is the answer but we can talk about that on another episode. I want to make sure that we talk about data because that’s such an important topic.
To your point about longevity and the democratization I’m talking about, think of who drives and what questions get asked in clinical trials. The reality is most of them are being driven by the industry for the purpose of approving a new drug or therapy. There is a certain amount of research in clinical trials that is done to inform policies. Those are largely led by nonprofit groups like ACC. In cardiology and oncology, it could be ASCO. It could be others.
That’s great, and they do great work but their scale is limited, especially if we’re using clinical trials to drive that because trials take a long time. They cost a lot of money. There’s a lot of work that goes into that. I’m all very clear that clinical trials and randomized double-blind placebo-control are the gold standards of evidence. It will be. It should be. I want our regulatory bodies to use it as a primary goal.
However, let’s say this out loud. If what I’m saying has now become possible, everybody should be able to ask questions. We don’t serve patients but I do get requests. If we’re talking about longevity, who is the group asking the observational research questions about longevity? It’s not that many people asking those questions. There’s Aubrey and others but why not ask more questions about that? Why not ask questions like Dylan Dodd did about existing drugs that might help with certain conditions?
If you think about the concept of longevity, probably what we’re talking about is not a new drug to cure longevity. Let’s explore Yamanaka factors but there are probably a lot of approved drugs or even non-prescription therapies that could help but because there’s no profit motive yet for that, there’s no funding for that research. What if it was cheap, fast, and easy? That’s what we’re creating.
Truly, as we have begun to expand this, we find our basis in peer review, transparency, and best-of-breed methodologies. We want to be the quality layer here because what people get concerned about using data is this. I can’t tell you how many times I heard somebody say, “Garbage in, garbage out.” I’m the first one to say this about healthcare data. It’s all garbage. We’re clear. None of it is good. It all has problems. The main thing though is can I identify and clearly tell you what those issues are? Can I correct them when that’s appropriate? Can I be transparent about that?
My feeling is observational data and real-world data is a term that gets used. It’s very useful. Here’s one of the things that our group published because of this topic. We said, “Let’s take 1,000 clinical trials that were run in the typical form. Let’s recreate them using real-world data. Let’s ask. How often did the studies have the same finding, the same direction, and the same degree?”
The answer to that was about 74% of the time, they completely agree. That’s pretty good but some might say, “What about when it didn’t agree?” We said, “Let’s also look at when you take a clinical trial and replicate it as a clinical trial.” We rerun trials sometimes with different sites and different patients, and it turns out they replicate each other at the same rate about 75% of the time.
In using real-world data or a clinical trial, we’re showing pretty much the same agreement. The differences though are important. Why did those 25% of the time disagree? Often, that is because we’re looking at subtly different groups of patients. Maybe they’re different demographically, or they have different histories. Maybe there were some differences in the way that the site of care administers care. These differences are critical. My whole point about all of this is that we should be using this data.
What we have built is a technology and service that opens that up, whether you’re a clinician with a question about an individual patient, somebody who’s trying to do some research, or even somebody curious, “Why are we holding back the ability to ask questions when there are so many good questions that need answering out there?” That’s what we are trying to democratize with Atropos.
Why are we holding back the ability to ask questions when there are so many good questions that need answering out there?
I love the idea of democratization. This is from my personal experience. It might be different for other people. The whole research hierarchy is very much an ivory tower. I went through a big research organization here and said, “I have this clinical question. This is not the only clinical question but I have these other clinical questions that I want to have answered. I don’t know how to answer them. You are a big research institution. How can we work together? I’m a professor at Tufts. I have all of these bona fides that allow me to be part of the conversation.”
This guy was sitting across from me. He’s like, “You need to learn how to fundraise. You need to go back and get an MPH or an MBA.” I was like, “There’s no way I’m going to do that. I’m not going to go and spend my time learning how to fundraise when I have all these other things that I have to do.” At least in my experience, it’s very much a difficult process to go through unless you devote your whole life to it as you did with a PhD.
Let me double-click on that. Let’s talk about what’s going on there. You go over there and say, “I would like to do this research.” There are three hurdles you hit immediately. Number one is they’re always worried about data security. They don’t want to give it to you because who knows who you’re going to give it to? We’re worried about HIPAA. Even if the data is de-identified, I don’t know who’s going to possess it. There’s an IT security layer.
The way we designed our solution is to remove that problem. No data is going anywhere. It stays where it is. The IT team can audit our application all you want. I’ve been through the Mayo Clinic audit and passed with flying colors. The bottom line is because we now have the cloud and we now have this compute layer, an application like this can work. I’ll install it locally. No data leaves. We will do an analysis but no patient-level data moves around. It’s better for everybody. It’s better for the institution, the IT group, and patients. You don’t worry about your data going anywhere. That’s hurdle one.
Let’s say you were to talk them into giving you access to the data in your case. We’re at hurdle two. Hurdle two is somebody, maybe you but probably not you, has to do the programming work and the statistical analysis work to answer your question. There’s probably another part, which is you have to make sure you’re asking a good question. It’s well-formed. It’s done with the right methods and all this stuff. This is why he said fundraising to you. You need to hire at least three people. You have a lab. Maybe you have people who can do that but if you don’t, lots of luck.
That’s where the funding comes in. Our platform specifically removes the need for those people while also making sure that the analysis is done with the best methods and making sure it’s transparent on how it was done and any bias that exists in the results. It’s almost like we’re standardizing that piece right there. What we find within academic institutions particularly is they will have a group. It’s usually called informatics. That might be 5 people or 100 people at some institutions. They’re the ones who are gating and in charge of keeping that quality, the methodology, and all of that.
When we talk to these groups, these are our best customers. Imagine you’re in that group. I was talking to a customer, and he was explaining, “I have a five-person team to do this.” I’m like, “How are you dealing with all the requests you get from clinicians like you? How are you dealing with it?” He’s like, “We say no.” I’m like, “What if we gave our tools to the informatics department? You can understand how it all works and be able to pull some of the strings yourself and use it to scale your team. What if I could 10X your five people? What would you do?”
He’s like, “I would open this up to this department because they have a ton of questions. I would let them ask questions. If they want us to staff part of this, we will do that.” We end up becoming this race car for the informatics team. In some institutions, that’s the way it needs to go. There are some institutions that have that function. They have informatics. They don’t want to unleash who knows what to every clinician. They still want to be able to gate it and control it. You will be the users. I’m fine with that.
Back to getting to questions, you’re meeting with that group already because you have a question. Let’s go to where the questions are if you’re coming to this group and enable them to be answered more quickly. Everything we build is to solve those problems right there. That’s a critical element to it. The last thing is I would love it if medical education was to the point where every clinician knew exactly how to design a well-structured study but they don’t. It’s hard. I have a PhD in this. It’s not that simple.
I would love it if medical education was to the point where every clinician knew exactly how to design a well-structured study, but they don’t and that’s hard.
One of the benefits of our service is if we’re dealing with a clinician who has an idea of these patients, we help design the study with them. They get to talk to a peer. They will ask a question and be like, “It’s all cancer patients with this history and these two drugs.” We will say something like, “Are you sure you want all cancer patients? Those cancers are different. Are you thinking more of breast cancer patients here?” They will say on an outcome, “I want to look at all skeletal-related events for these patients.” We will be like, “What do you mean by skeletal-related events? Do you mean hypercalcemia, malignancy, or death? Do you mean intervention with a bone-modifying agent? Do you mean spinal fractures? What are the things you mean?”
We maintain a central library and database of what we call phenotypes, which are the best ways to define all of those things and the most appropriate settings. We have a whole repository of that. We can say, “This is the way most of the people asking a question like this did it. Would you like to do it that way?” The clinician who has an observation has an idea but maybe not a researcher. We can help them get to a well-formed question and then apply the appropriate statistical methodologies.
Normally, with Atropos and the Green Button service, we have a clinician sitting on the other side. We’re in the world of ChatGPT, LLMs, and AI. We looked at that at first and said, “That’s a little bit scary because quality and error rate are important to us. If we’re going to influence policy or even an individual patient’s care, we want to be sure that there’s no funkiness going on.”
We published a paper in the spring where we took 100 questions we got from doctors and researchers and ran it through ChatGPT-4 to see what it would say. Your audience can read this paper. It’s out of the Human-Centered AI group at Stanford. The headline was, “ChatGPT could only answer the questions about 40% of the time.” Do you want to know why? It would say, “There’s not enough clinical trial evidence out there to answer this question,” which is my whole point. We need more evidence.
We need more evidence.
The other problem was it hallucinated about 9% of the time, which would not be acceptable to us. We took this finding and said, “There’s something to these LLMs. We know something about it.” ChatGPT-4 is the fastest-growing website of all time. People like the user experience of it. It does look like in some cases, it’s good at automating human steps. It’s being used all over the place now to do that. We say, “What about us? We have a clinician who’s talking to this other clinician. Could we replace parts of that with an LLM while also keeping our cortex separate, the one that does the accuracy and the analysis?”
We launched ChatRWD, which stands for Chat Real-World Data. A user without any help will go in and ask a question. It is a prompt-based interface, a chatbot. What it will do is it will help extract the question they’re trying to ask. It will detect, “This is what you’re asking. Is this right, yes or no? Do you want suggestions on how to improve this question?” It will give you suggestions. You can adopt those.
It will even go as far as helping you choose the definitions, “How are we going to define the history of diabetes? Maybe it’s two ICD codes showing the diagnosis. Maybe the HbA1c is over 45. Maybe if they’re on insulin, we will say they have diabetes.” It will help you choose that definition based on what we know to be the right definition. Users can say, “That’s my question.” They hit submit. In under three minutes, while they’re still in the chat, we will produce that whole study fully automated for them.
I don’t even know what this is yet because study production normally takes months. We have to hire these people. What if I can give a tool like ChatRWD to researchers and maybe even some data clinicians? They can be generating this evidence on the fly. We’re in beta, and the things people are doing with it are wild. What we’re able to do is have an LLM-based experience people like while ensuring accuracy, not bringing the hallucination to play. We will be publishing that in 2024.
I don’t know if you saw Elon Musk’s interview on DealBook. The big headline was that he told his advertisers to go F off, which I thought was hilarious. One of the things that he also said was that he doesn’t think that AI will ever be able to realistically attain the same level of intelligence that a number of experts sitting together will be able to attain, a lot of humans sitting down.
That’s what I look at with the amount of information that’s available in the electronic medical record. All of these experts have written about the patient that they saw right in front of them. If we can coordinate that with something like yourself or some other way of accumulating all that brain power of humans into some machine or tool, that is going to be much more powerful than artificial intelligence. This has been so interesting.
Let me respond to that. First of all, I’m lucky enough to have co-founders like Nigam Shah and Saurabh. We have super talent on this topic. I would encourage everybody to read a lot of the work being produced by Nigam at Stanford and the Human-Centered AI group. Here’s the thing. Why are we all talking about all this stuff? It’s because the cloud layer exists, and the compute layer is significant. This is math that has been around for a while.
What’s exciting and scary sometimes is with all this scale, where does this thing go? Our experience is that primarily, it’s a human augmentation tool. If you think about what I said at ChatRWD, what is the AI doing? It’s helping you clarify your thoughts a little bit. There are areas that LLMs or other AI techniques are particularly good at but it’s always being deployed. In our case, humans on our team built things to deploy to help a user experience. It’s not being tasked with becoming this super knowledge base.
I tend to agree with Elon. I’m not saying it’s a zero risk that something like that happens. I do think the work being done in OpenAI is as close as it comes to some of that stuff but I don’t think we’re there yet. There are still many human checkpoints throughout it. In terms of intelligence, my colleague Morgan Cheatham had ChatGPT take the USMLE exam, pass it, and all this stuff. We always had these magic bars, “It passed the medical exam. It’s as smart as a human.” I’m like, “It’s smart at passing the medical exam. That’s something different.”
It’s how it’s being deployed, the checks, and stuff that is going in. We’re all about transparency and how this stuff is used. We’re a long way off from making decisions. I’m not hearing from doctors that they want somebody doing their job. I’m hearing from them, “We want augmentation to the stuff that we do like the stuff being done by companies like Abridge. Document my meeting with a patient, pull the appropriate records and summarization, and save me from doing that.”
My wife is a physician. She shouldn’t have to scroll to page 83 of a note in Epic. She should be able to type something, and it pulls back the relevant information. That’s a great use of this technology. That is not an AI doctor treating you. That’s not what it is. It’s exciting because there are a ton of areas for optimization, whether it’s me automating research or whether it’s document retrieval and search. Tons of great stuff are going to happen from this but it is non-zero that it’s dangerous. We need to be diligent and focus on transparency for that.
Honestly, this was an interesting and exciting conversation. I appreciate you coming to the show. I end my show with the same three questions with every single guest. We’re going to end with those. Where do you get your inspiration from? For me, it’s science fiction. I look at the utopian visions of science fiction, whether it’s Star Trek or Isaac Asimov. Those are the futures that I want to live in. I look at those, and I want to be inspired by them because I want to build those futures both for my patients and my kids. Where do you gain your inspiration from?
I love all that stuff too. I consume now more through my ears. Whether it’s audiobooks or podcasts, I consume a lot. I think a lot about history and where we are in that process. On the sci-fi side, I’m postulating about what our future could be. Elon is a very inspiring guy and a lot of that. It takes a lot of self-realization to understand why you do anything. That’s a journey.
I lost my mother to cancer when I was fourteen. If I think about the things I’ve done, it has been chasing that cure and that impact, “I’m a chemist. I’m going to make a drug and save my mother. I’m a PhD pharmacologist. I’m going to discover something at the bench or even data.” Any pursuit becomes an obsession. I’m angry about it in a way, “Why can’t this be solved? Why can’t we save that person? The data is there. We have all these tools. What is stopping us?”
The urgency that wakes me up in the morning is, “We’re still not there yet we have everything.” It’s up to folks like ourselves and others to chase that down. To get there, you can say that. Cancer touches everybody. I’m not unique but you have to let curiosity drive you down that path, “Why isn’t it solved? Why not?” You have to chase that down. That’s what drives me every single day to push what we’re doing at Atropos but also to find the answer to this stuff. That’s what we’re all trying to do.
You have to let curiosity drive you down that path toward a solution.
I appreciate that. I lost both my parents to cancer too. I understand that drive. It’s something that I deal with every day. All the best of luck to you with Atropos and all of the stuff that you’re doing. Where do you see this field in ten years? We touched on so many different fields but where do you see that happening?
I’ll give a very Homer perspective on this. If I’m right about what we’re trying to do at Atropos Health, what does that mean? That means every decision could have personalized evidence for that patient. What effect does that end up having? It could be revolutionary for the practice of medicine because if you give physicians the right evidence and information, they can make great decisions. This allows for a better virtualization of care and makes a doctor’s life easy.
On some level, it could become the days of old where the doctor can make house calls. It would be virtual telecalls now. What if we could hand the power back to physicians by giving them this evidence power and letting them take that rule again? You talk about the human with the AI as the one who applies this information. That’s interesting. Being married to a clinician and knowing many clinicians, that would be a great change for them because the thing that they’re working on is very cog-in-a-wheel at times. It’s built around these business structures, and it’s gotten so far away from why a lot of people wanted to practice.
I hope that we can not only generate all this evidence for patients but it can change the way physicians practice. The rest of the world globally is in a position to even start from a better place with all this. They don’t have the historic infrastructure we do. What if we could jump them ahead years and decades by doing this and allow for evidence on their populations? There’s not a lot of evidence in some of those countries. My hope is that it can not only change the way we make decisions for individual patients but also change the way we practice.
I agree with the idea that all of the admin work makes you feel like a cog in the wheel. I’m sure that your wife would agree with the idea of the AI revolution. Making that go away would be huge for us. I can’t wait until that happens. That’s interesting. Last question, we talked about so many different technologies and topics.
Of all of the stuff that you’re reading, whether it’s science, technology, or all this stuff that you’re keeping up with, what are you most excited about for the future, your children, and the next generation? I always tell all my guests that I can’t wait until we have personalized humanoid robots. I can’t wait until I don’t have to wash the dishes anymore, and I can tell the robot to do it. What about you? What are you most excited about?
You could argue we already are androids by some definition with the cell phones we carry in our pockets. What’s exciting about this AI stuff and ChatGPT is if you think about human creativity, which is the thing that AI could never capture, and some people would argue about this, we are being enabled now with a set of tools. I think about how my kids could have an idea to do something and be able to execute it with the tools around them. That’s the beginning of where that could go.
In a vein adjacent to this, I’m interested in AR and VR, particularly AR or augmented reality. That’s coming at us quickly. That’s going to change a whole bunch of stuff and talk to your dishwasher but beyond that, there’s new stuff out now like gesturing. Do you remember before we had Google Maps what it was like driving? All of a sudden, we had Google Maps. It completely changed the way we interface with the physical world in a way. This is going to go a step further because now, you have a digital interface to physical equipment and your environment. That’s soon too, and I’m interested in where that goes.
I told my wife that I was going to be one of the first people who buy the new Apple augmented reality setup. I can’t wait for that too. Thank you so much for joining us, Brigham. It was a pleasure. We talked about a lot of cool and interesting things. Thank you so much to our audience. For those who read on a regular basis, we will see you in the future. Have a great day, everybody.
Thank you.
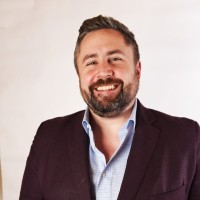
Brigham Hyde
CEO - Atropos Health
Dr. Brigham Hyde is CEO and co-founder of Atropos Health since August 2022. He provided funding and support for Atropos Health’s official launch in late 2020. Hyde has a significant track record of building businesses in the health tech and real-world data (RWD) space and most recently served as President of Data & Analytics at Eversana.
Prior to that role, Mr. Hyde served as a healthcare partner at the AI venture fund Symphony AI, where he led the investment in, co-founded, and operated Concert AI, an oncology RWD company – most recently valued at $1.9B. Hyde held previous roles as Chief Data Officer at Decision Resources Group, which was acquired by Clarivate for $900M in 2020. He has also served on the Global Data Science Advisory Board for Janssen, as a research faculty at MIT Media Lab, and served as adjunct faculty at Tufts Medical School.